Descriptive Data Mining vs. Predictive Data Mining — What's the Difference?

By Tayyaba Rehman — Published on January 6, 2024
Descriptive data mining summarizes past data to understand what has happened, while predictive data mining uses the data to forecast future outcomes.
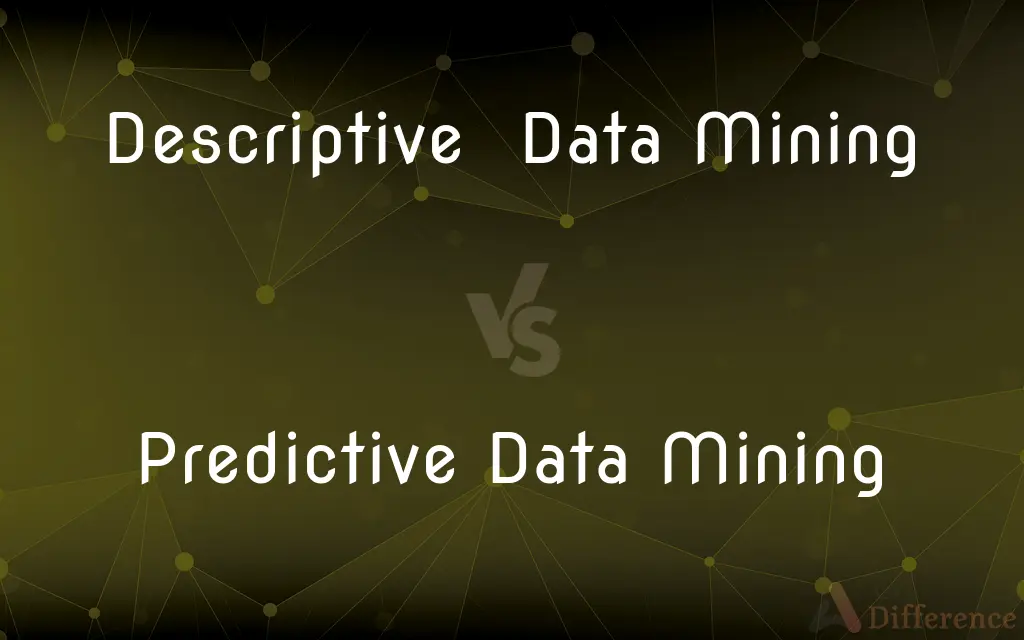
Difference Between Descriptive Data Mining and Predictive Data Mining
Table of Contents
ADVERTISEMENT
Key Differences
Descriptive data mining is focused on parsing historical data to gain an understanding of the patterns and relationships that have occurred within it. It is akin to a detailed summary, providing insights into various aspects of the data without forecasting future events.
Predictive data mining, by contrast, uses algorithms to model the likelihood of future events and trends based on historical data. It's a step beyond description, aiming to anticipate what will happen next based on patterns identified in the data.
In descriptive data mining, data scientists may employ techniques such as clustering and association to identify patterns that explain the behavior or profile of the data. It’s about understanding the data’s story up to the current moment.
Predictive data mining employs different algorithms, such as classification and regression, which take the analysis further to make predictions about future actions, trends, or behaviors. The focus here is not just on understanding, but on forecasting.
Both descriptive and predictive data mining are integral to business intelligence. Descriptive mining helps organizations understand their current situation, while predictive mining helps them plan for the future by forecasting trends and behaviors.
ADVERTISEMENT
Comparison Chart
Objective
To summarize past data and find patterns.
To predict future events based on past data.
Techniques Used
Clustering, association.
Classification, regression, time series analysis.
Output
Reports, summaries, dashboards.
Predictive models, forecasts, risk assessments.
Focus
Understanding what happened.
Anticipating what will happen.
Data Utilization
Analyzes past data for insights.
Uses past data to build predictive models.
Compare with Definitions
Descriptive Data Mining
Uncovers patterns from historical data.
Descriptive data mining revealed that our peak sales occur on weekends.
Predictive Data Mining
Estimates future outcomes based on historical data.
Predictive data mining forecasted a rise in demand for our products.
Descriptive Data Mining
Involves techniques like clustering to identify groups within data.
Clustering in descriptive data mining grouped customers by purchasing habits.
Predictive Data Mining
Involves building models for prediction and classification.
Predictive data mining classified customers' future buying potential.
Descriptive Data Mining
Focuses on summarization and understanding of past data.
Using descriptive data mining, the company could see seasonal buying trends.
Predictive Data Mining
Often employs regression techniques for forecasting.
A regression model from predictive data mining estimated next month's traffic.
Descriptive Data Mining
Utilizes association rules to discover relationships.
Descriptive data mining found an association between weather and product sales.
Predictive Data Mining
Assists in risk assessment and decision-making.
Predictive data mining reduced risk by predicting loan defaulters.
Descriptive Data Mining
Aims to provide a clear picture of past business performance.
Descriptive data mining helped us understand the sales dip in the last quarter.
Predictive Data Mining
Utilizes algorithms to predict future trends.
We used predictive data mining to forecast next year's sales trends.
Common Curiosities
Can descriptive data mining predict future trends?
No, it focuses only on summarizing past data, not on future predictions.
What are common tools used for descriptive data mining?
Tools like Tableau and Power BI are used for visualization and summarization.
How does predictive data mining work?
It analyzes historical data to build models that predict future outcomes.
Can descriptive data mining help in improving customer satisfaction?
Yes, by understanding past customer behavior and preferences.
Is machine learning a part of predictive data mining?
Yes, many predictive models use machine learning algorithms.
What statistical methods are common in predictive data mining?
Regression analysis, decision trees, and neural networks.
Does descriptive data mining require a large amount of data?
It can work with various data sizes but larger datasets can provide more insights.
What is the goal of descriptive data mining?
To interpret and summarize historical data to understand patterns.
How is predictive data mining used in finance?
It's used to predict stock trends, credit risks, and investment outcomes.
Is predictive data mining foolproof?
No, predictions are probabilistic and carry a degree of uncertainty.
What industries benefit from predictive data mining?
Finance, healthcare, marketing, retail, and many more.
How accurate is predictive data mining?
Accuracy can vary widely and depends on data quality and modeling approach.
Can predictive data mining use real-time data?
Yes, especially for dynamic models that adjust to new data.
Are there privacy concerns with descriptive data mining?
Yes, especially if it involves sensitive or personal data.
Can I use both descriptive and predictive data mining together?
Yes, they complement each other for comprehensive data analysis.
Share Your Discovery
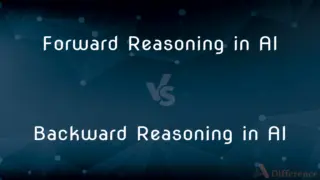
Previous Comparison
Forward Reasoning in AI vs. Backward Reasoning in AI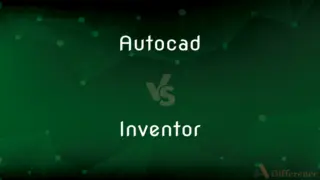
Next Comparison
Autocad vs. InventorAuthor Spotlight

Written by
Tayyaba RehmanTayyaba Rehman is a distinguished writer, currently serving as a primary contributor to askdifference.com. As a researcher in semantics and etymology, Tayyaba's passion for the complexity of languages and their distinctions has found a perfect home on the platform. Tayyaba delves into the intricacies of language, distinguishing between commonly confused words and phrases, thereby providing clarity for readers worldwide.