Homoscedasticity vs. Heteroscedasticity — What's the Difference?

Edited by Tayyaba Rehman — By Urooj Arif — Updated on March 17, 2024
Homoscedasticity implies constant variance across a dataset, while heteroscedasticity indicates variance changes.
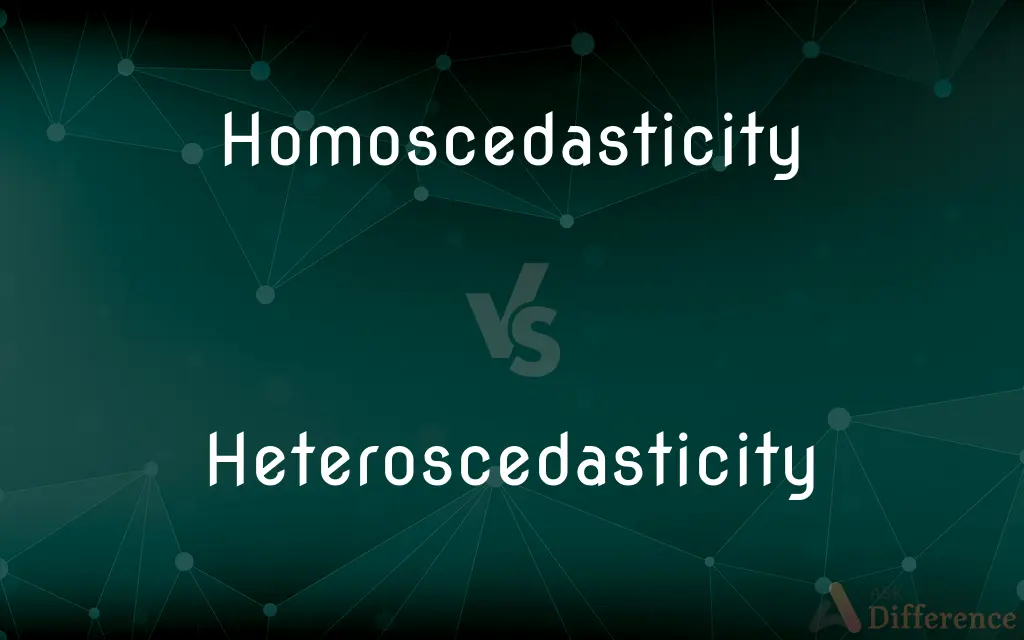
Difference Between Homoscedasticity and Heteroscedasticity
Table of Contents
ADVERTISEMENT
Key Differences
Homoscedasticity is a fundamental assumption in many statistical models, implying that the variance within a dataset is constant across all levels of an explanatory variable. Whereas heteroscedasticity refers to the presence of varying variances within a dataset across different levels of an explanatory variable, challenging the validity of some statistical analyses.
In the context of regression analysis, homoscedasticity suggests that the residuals (differences between observed and predicted values) are evenly spread throughout the range of predicted values. On the other hand, heteroscedasticity in regression analysis is identified when residuals do not spread evenly but increase or decrease in variance with the level of an independent variable or as a function of the predicted values.
Detecting homoscedasticity often involves visual inspection of residual plots, where a random, consistent spread of residuals suggests homoscedasticity. Conversely, patterns in residual plots, such as a funnel shape widening or narrowing, indicate heteroscedasticity, signaling the need for adjustments in the analysis.
Addressing homoscedasticity in statistical models ensures the reliability of standard errors, confidence intervals, and significance tests, which are crucial for hypothesis testing. In contrast, heteroscedasticity can be managed through transformations of variables, adopting robust standard errors, or using models designed to handle varying variances, like generalized least squares.
In terms of impact, homoscedasticity contributes to the precision and reliability of statistical estimates, making model predictions more trustworthy. Heteroscedasticity, if unaddressed, can lead to inefficient estimates and biased standard errors, potentially misleading conclusions from statistical tests.
ADVERTISEMENT
Comparison Chart
Definition
Constant variance across a dataset
Varying variance across a dataset
Importance in Analysis
Assumption for linear regression and other models
Violation leads to need for model adjustment
Detection Methods
Residual plots, lack of patterns
Residual plots with patterns (e.g., funnel shape)
Implications
Reliable standard errors, confidence intervals
Biased standard errors, misleading statistical tests
Solutions
Standard analysis methods applicable
Variable transformations, robust standard errors
Compare with Definitions
Homoscedasticity
A prerequisite for certain statistical analyses ensuring accurate hypothesis testing.
Before proceeding with the analysis, we checked for homoscedasticity to validate our model's assumptions.
Heteroscedasticity
Often requires remedial measures to correct or accommodate the variance in the analysis.
We used logarithmic transformation to address the heteroscedasticity observed in our data.
Homoscedasticity
Uniform variance of residuals across the range of predicted values.
A linear regression model displaying a scatterplot of residuals with a constant spread indicates homoscedasticity.
Heteroscedasticity
Indicates potential problems with regression assumptions, affecting the model's validity.
Detecting heteroscedasticity led us to reconsider our model's assumptions and seek alternative methods.
Homoscedasticity
Detected through visual inspection or statistical tests, looking for a consistent spread of residuals.
The plot of residuals revealed homoscedasticity, supporting the use of conventional statistical tests.
Heteroscedasticity
Unequal variance of residuals across the range of predicted values.
The widening spread of residuals in our regression analysis signaled heteroscedasticity.
Homoscedasticity
Maintains the effectiveness of standard statistical methods without requiring adjustments.
Given the homoscedastic nature of our data, we applied standard linear regression techniques confidently.
Heteroscedasticity
Can be indicative of underlying issues with data or model specification.
The presence of heteroscedasticity suggested that our model might not be capturing all relevant variables.
Homoscedasticity
Associated with stability and reliability in model predictions.
Homoscedasticity in our dataset assured us of the robustness of our predictive model.
Heteroscedasticity
Managed through techniques such as transforming variables or using robust error estimates.
To mitigate the effects of heteroscedasticity, we adopted robust standard errors in our analysis.
Homoscedasticity
In statistics, a sequence (or a vector) of random variables is homoscedastic if all its random variables have the same finite variance. This is also known as homogeneity of variance.
Heteroscedasticity
In statistics, a vector of random variables is heteroscedastic (or heteroskedastic; from Ancient Greek hetero "different" and skedasis "dispersion") if the variability of the random disturbance is different across elements of the vector. Here, variability could be quantified by the variance or any other measure of statistical dispersion.
Homoscedasticity
(statistics) A property of a set of random variables such that each variable has the same finite variance.
Heteroscedasticity
(statistics) The property of a series of random variables of not every variable having the same finite variance
Common Curiosities
What is homoscedasticity important for in statistical analysis?
Homoscedasticity is crucial for the validity of hypothesis tests, ensuring that standard errors and confidence intervals are accurate.
Why does heteroscedasticity pose a problem for regression analysis?
It can lead to biased estimates of standard errors, affecting the reliability of hypothesis testing and confidence intervals.
How can I detect heteroscedasticity in my data?
Heteroscedasticity can be detected through visual inspection of residual plots or by using statistical tests designed to identify variance inconsistencies.
Is heteroscedasticity more common in certain types of data?
Yes, it is often more prevalent in datasets with a wide range of values or where the variance increases with the size of the measurement.
Are there specific tests to detect homoscedasticity?
Tests like the Breusch-Pagan or White test are specifically designed to detect heteroscedasticity, indirectly confirming homoscedasticity if not found.
Can transforming my data always solve heteroscedasticity?
While transformations can help, they may not always completely solve heteroscedasticity, and other methods like robust standard errors might be necessary.
Can homoscedasticity be assumed in all regression models?
No, homoscedasticity is an assumption that needs to be tested, as many real-world datasets exhibit heteroscedasticity.
What are some common methods to address heteroscedasticity?
Methods include transforming the dependent variable, using weighted least squares, or applying robust standard errors.
Can a dataset exhibit both homoscedasticity and heteroscedasticity?
A dataset might exhibit areas of both, but typically, it's classified based on the prevailing pattern affecting the analysis.
What happens if I ignore heteroscedasticity in my analysis?
Ignoring heteroscedasticity can result in misleading statistical inferences, such as incorrect rejection of null hypotheses.
How does homoscedasticity affect model selection in statistics?
Homoscedasticity supports the use of simpler models like ordinary least squares regression, as more complex models may not be needed to handle variance issues.
How does sample size impact the detection of heteroscedasticity?
Larger sample sizes can make it easier to detect heteroscedasticity, as patterns in variance become more apparent.
Share Your Discovery

Previous Comparison
Concerto vs. Concert
Next Comparison
Scarce vs. ScarcelyAuthor Spotlight

Written by
Urooj ArifUrooj is a skilled content writer at Ask Difference, known for her exceptional ability to simplify complex topics into engaging and informative content. With a passion for research and a flair for clear, concise writing, she consistently delivers articles that resonate with our diverse audience.

Edited by
Tayyaba RehmanTayyaba Rehman is a distinguished writer, currently serving as a primary contributor to askdifference.com. As a researcher in semantics and etymology, Tayyaba's passion for the complexity of languages and their distinctions has found a perfect home on the platform. Tayyaba delves into the intricacies of language, distinguishing between commonly confused words and phrases, thereby providing clarity for readers worldwide.