Parametric Test vs. Nonparametric Test — What's the Difference?

Edited by Tayyaba Rehman — By Fiza Rafique — Published on December 10, 2023
Parametric Tests assess means using assumptions about population parameters. Nonparametric Tests are distribution-free and make fewer assumptions.
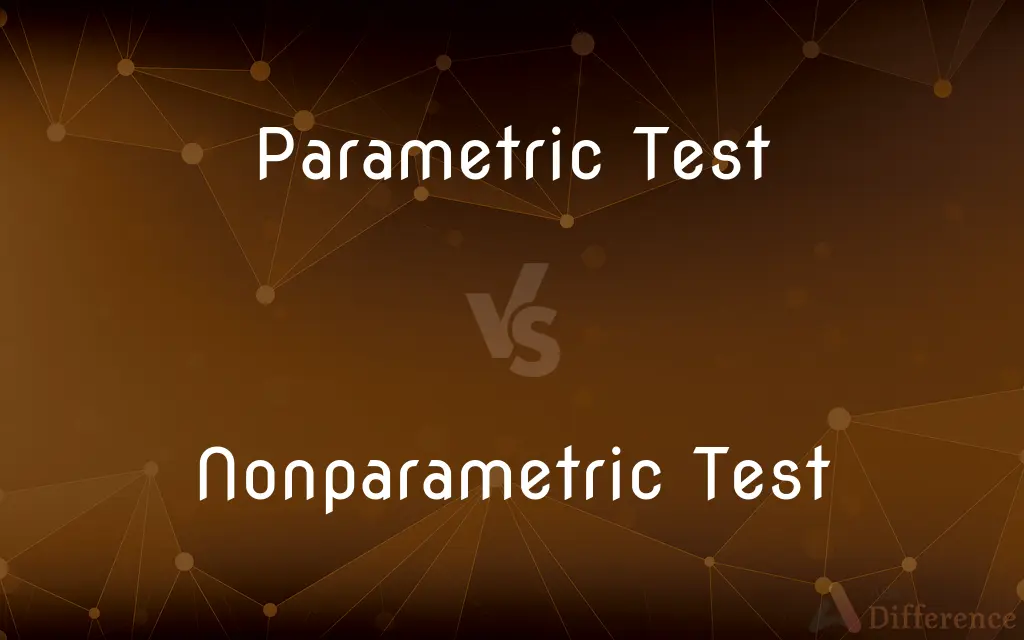
Difference Between Parametric Test and Nonparametric Test
Table of Contents
ADVERTISEMENT
Key Differences
Parametric Tests and Nonparametric Tests are both essential tools in statistical analysis. However, while Parametric Tests are based on specific population parameter assumptions, Nonparametric Tests are more flexible and make fewer assumptions about the population's distribution.
A defining characteristic of Parametric Tests is that they assume underlying statistical distributions. They are often used when the data is normally distributed. On the other hand, Nonparametric Tests, often termed distribution-free tests, are used when the data doesn't meet these normality criteria.
The power of a Parametric Test is typically higher when its assumptions are met, which means it can detect a significant effect more efficiently. In contrast, a Nonparametric Test, because of its broader applicability, might have less power but is more versatile in handling various types of data.
Examples provide a clearer understanding. The t-test, a Parametric Test, is used to compare the means of two groups. On the other hand, the Mann-Whitney U test, a Nonparametric Test, is used for the same purpose but without assuming normality in the data.
To summarize, while Parametric Tests hinge on specific distributional assumptions and assess means, Nonparametric Tests are more flexible, offering analysis when these assumptions are not met.
ADVERTISEMENT
Comparison Chart
Assumptions
Assumes normal distribution
Makes fewer assumptions
Data Type
Interval or ratio
Ordinal, nominal, interval, or ratio
Test Power
Higher when assumptions are met
Typically less powerful but more versatile
Common Examples
T-test, ANOVA
Mann-Whitney U, Kruskal-Wallis
Sensitivity to Outliers
Sensitive
Less sensitive
Compare with Definitions
Parametric Test
Examines population parameters, typically means.
The t-test is a Parametric Test for assessing differences in means.
Nonparametric Test
A test that doesn't require distributional assumptions.
The Kruskal-Wallis is a Nonparametric Test comparing medians.
Parametric Test
Often assumes data normality.
Before using a Parametric Test like ANOVA, data normality should be checked.
Nonparametric Test
Provides flexibility in statistical analysis.
Nonparametric Tests, like Spearman's rank correlation, offer versatility.
Parametric Test
Sensitive to outliers.
Extreme scores can affect the outcome of a Parametric Test.
Nonparametric Test
More robust against outliers.
A Nonparametric Test is often chosen when outliers are present.
Parametric Test
A statistical test with specific distributional assumptions.
The ANOVA is a Parametric Test used to compare means across multiple groups.
Nonparametric Test
Often used when data isn't normally distributed.
If data isn't normal, a Nonparametric Test like Mann-Whitney might be apt.
Parametric Test
Requires data to be interval or ratio.
Regression analysis, a Parametric Test, needs interval or ratio data.
Nonparametric Test
Suitable for all data types, including ordinal and nominal.
The Chi-square test, a Nonparametric Test, works with nominal data.
Common Curiosities
Are Nonparametric Tests limited to non-normal data?
No, they can be used for any data but are especially useful when normality isn't assumed.
Can Parametric Tests handle outliers well?
No, Parametric Tests are typically sensitive to outliers.
Why are Parametric Tests called 'parametric'?
Because they make assumptions about population parameters.
When should one opt for a Nonparametric Test?
Nonparametric Tests are chosen when data doesn't meet the assumptions of Parametric Tests.
Are Parametric Tests always based on means?
Mostly yes, but they primarily focus on population parameters, often means.
Can a Parametric Test be used for binary data?
No, binary data is better suited for Nonparametric Tests.
What type of data suits a Parametric Test?
Interval or ratio data that is normally distributed is ideal for a Parametric Test.
Are Nonparametric Tests always the best choice for ordinal data?
Typically, yes. Nonparametric Tests are well-suited for ordinal data.
Is the Mann-Whitney U test a Parametric Test?
No, it's a Nonparametric Test.
Which test is more powerful: Parametric or Nonparametric?
Parametric Tests are generally more powerful when their assumptions are met.
Can Nonparametric Tests be used for large sample sizes?
Yes, Nonparametric Tests can be used regardless of sample size.
What happens if data isn't normally distributed but a Parametric Test is applied?
The results might be invalid or misleading.
Which is more versatile: Parametric or Nonparametric Tests?
Nonparametric Tests, due to fewer assumptions, are generally more versatile.
Why use a Nonparametric Test when Parametric Tests are more powerful?
Because the data might not meet the assumptions of Parametric Tests.
Can transformations make non-normal data suitable for Parametric Tests?
Sometimes, transformations like logarithms can normalize data, making it suitable for Parametric Tests.
Share Your Discovery
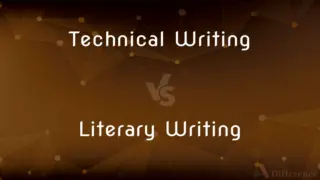
Previous Comparison
Technical Writing vs. Literary Writing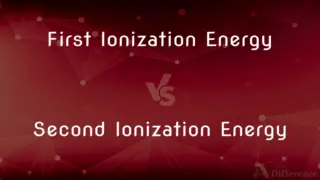
Next Comparison
First Ionization Energy vs. Second Ionization EnergyAuthor Spotlight

Written by
Fiza RafiqueFiza Rafique is a skilled content writer at AskDifference.com, where she meticulously refines and enhances written pieces. Drawing from her vast editorial expertise, Fiza ensures clarity, accuracy, and precision in every article. Passionate about language, she continually seeks to elevate the quality of content for readers worldwide.

Edited by
Tayyaba RehmanTayyaba Rehman is a distinguished writer, currently serving as a primary contributor to askdifference.com. As a researcher in semantics and etymology, Tayyaba's passion for the complexity of languages and their distinctions has found a perfect home on the platform. Tayyaba delves into the intricacies of language, distinguishing between commonly confused words and phrases, thereby providing clarity for readers worldwide.