Type I Error vs. Type II Error — What's the Difference?

Edited by Tayyaba Rehman — By Fiza Rafique — Published on October 25, 2024
Type I Error occurs when a true null hypothesis is incorrectly rejected; Type II Error happens when a false null hypothesis fails to be rejected.
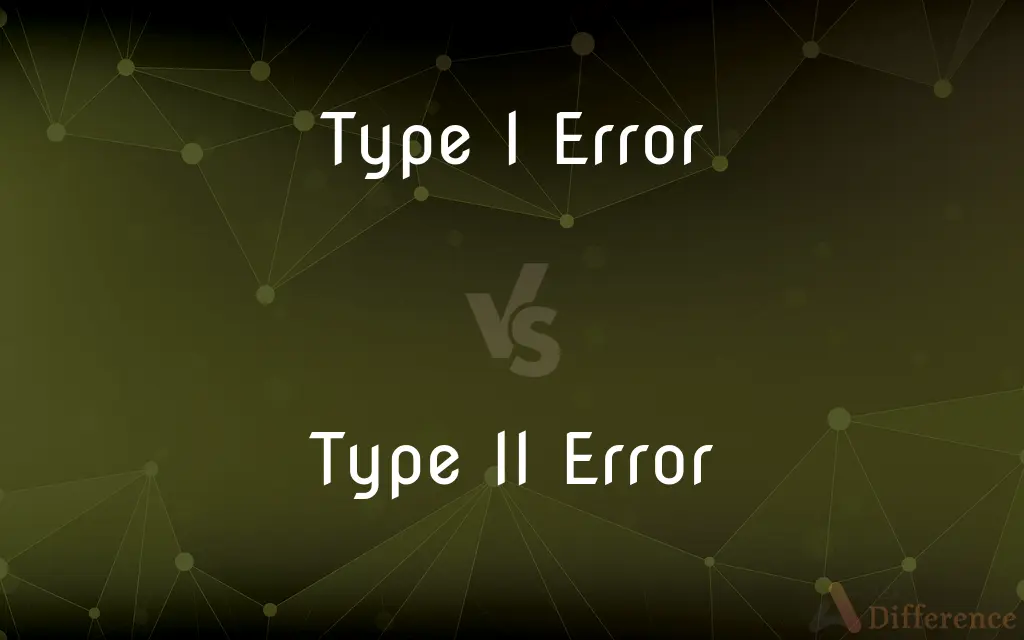
Difference Between Type I Error and Type II Error
Table of Contents
ADVERTISEMENT
Key Differences
Type I Error, also known as a "false positive," involves rejecting a true null hypothesis, indicating a belief that there is an effect or difference when there isn't one. On the other hand, Type II Error, or a "false negative," occurs when a false null hypothesis is not rejected, suggesting that no effect or difference was found when, in fact, there was.
Type I Errors are influenced by the significance level (α), the threshold for deciding when to reject the null hypothesis. Lowering α reduces the risk of a Type I Error. Whereas, Type II Errors are associated with the power of a test (1−β), where β is the probability of a Type II Error occurring. Increasing the sample size or effect size can enhance the power, thereby reducing the likelihood of a Type II Error.
The consequences of Type I and Type II Errors differ depending on the context. A Type I Error might lead to unnecessary alarm or actions, such as treating a disease that is not present. Conversely, a Type II Error could result in missed opportunities for intervention, like failing to treat a disease when it is actually present.
Managing these errors involves trade-offs, as decreasing the probability of one error often increases the risk of the other. Researchers choose significance levels and design studies to balance these risks based on the relative importance of avoiding each type of error in their specific context.
Comparison Chart
Definition
Rejecting a true null hypothesis
Failing to reject a false null hypothesis
ADVERTISEMENT
Also Known As
False positive
False negative
Influenced By
Significance level (α)
Power of the test (1−β)
Consequences
Unnecessary alarm/actions
Missed opportunities for intervention
Management Strategy
Lower α to reduce risk
Increase sample size/power to reduce risk
Compare with Definitions
Type I Error
Occurs when the evidence suggests an effect that does not exist.
Concluding a new drug works when it doesn't.
Type II Error
Influenced by the power of the test (1−β).
A higher sample size decreases the risk of a Type II Error.
Type I Error
Determined by the significance level (α).
Setting α at 0.05 means a 5% risk of a Type I Error.
Type II Error
Represents a missed detection.
Overlooking a true fire alarm.
Type I Error
Represents a false alarm.
Acting on a fire alarm when there's no fire.
Type II Error
Occurs when the evidence fails to detect an actual effect.
Concluding a new drug is ineffective when it is effective.
Type I Error
An error made by incorrectly rejecting a true null hypothesis.
Diagnosing a healthy person with a disease.
Type II Error
An error made by failing to reject a false null hypothesis.
Not detecting a disease in an ill person.
Type I Error
Avoided by requiring stronger evidence to reject the null hypothesis.
Using a lower α level for critical decisions.
Type II Error
Avoided by designing more powerful studies.
Increasing sample size or the effect size.
Common Curiosities
How can you reduce Type I Errors?
By setting a lower significance level (α) for the hypothesis test.
How can Type II Errors be minimized?
By increasing the sample size or the effect size, thus enhancing the test's power.
What is a Type I Error?
Rejecting a true null hypothesis, also known as a false positive.
Which is worse, Type I or Type II Error?
It depends on the context; for critical health decisions, a Type I Error might be more severe, while in research settings, a Type II Error might be more detrimental.
What is a Type II Error?
Failing to reject a false null hypothesis, also known as a false negative.
Does increasing sample size affect Type I Error?
No, the sample size primarily affects the power of the test and, thus, the Type II Error rate.
Can both Type I and Type II Errors occur simultaneously?
They cannot occur simultaneously for the same hypothesis test; a decision either results in one type of error or is correct.
Is Type I Error related to the p-value?
Yes, a p-value lower than the significance level (α) can lead to rejecting the null hypothesis, potentially causing a Type I Error.
What role does power (1−β) play in Type II Error?
The power of a test is the probability of correctly rejecting a false null hypothesis, inversely related to the risk of a Type II Error.
Can you control for both Type I and Type II Errors simultaneously?
Yes, but improving one often worsens the other, requiring a balance based on study priorities.
How does the significance level (α) affect Type I Error?
The significance level directly sets the probability of making a Type I Error; a lower α means a lower risk.
How does effect size influence Type II Error?
Larger effect sizes make it easier to detect an effect, thus reducing the likelihood of a Type II Error.
Why is it impossible to eliminate both Type I and Type II Errors completely?
Because reducing the probability of one increases the probability of the other, and absolute certainty is unattainable in hypothesis testing.
How do researchers choose between α and β?
They balance the two based on the relative importance of avoiding each type of error in their specific study context.
What does a high power (1−β) indicate?
A high power indicates a high probability of detecting an effect if there is one, minimizing the risk of a Type II Error.
Share Your Discovery
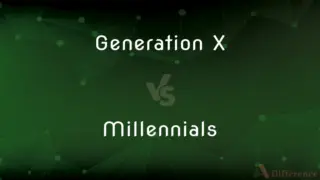
Previous Comparison
Generation X vs. Millennials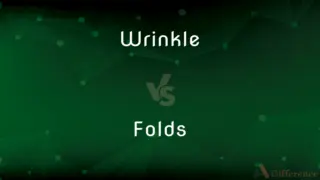
Next Comparison
Wrinkle vs. FoldsAuthor Spotlight

Written by
Fiza RafiqueFiza Rafique is a skilled content writer at AskDifference.com, where she meticulously refines and enhances written pieces. Drawing from her vast editorial expertise, Fiza ensures clarity, accuracy, and precision in every article. Passionate about language, she continually seeks to elevate the quality of content for readers worldwide.

Edited by
Tayyaba RehmanTayyaba Rehman is a distinguished writer, currently serving as a primary contributor to askdifference.com. As a researcher in semantics and etymology, Tayyaba's passion for the complexity of languages and their distinctions has found a perfect home on the platform. Tayyaba delves into the intricacies of language, distinguishing between commonly confused words and phrases, thereby providing clarity for readers worldwide.